Deep Learning for Inverse Design of Complex Nanoparticle Heterostructures
2024-12-26
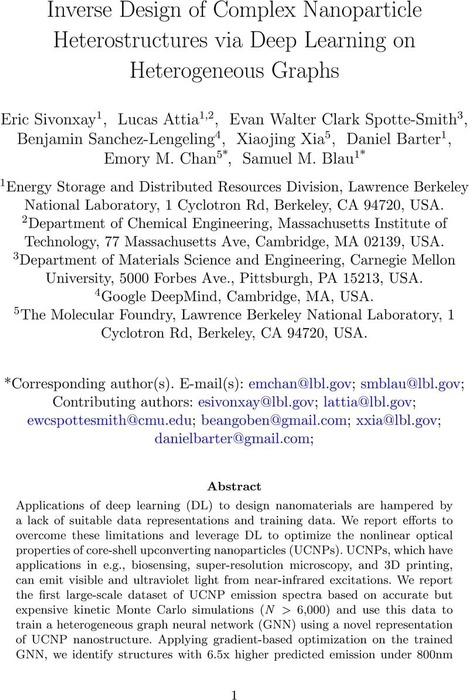
Researchers used deep learning and heterogeneous graph neural networks to inverse design upconverting nanoparticles (UCNPs) with applications in biosensing, super-resolution microscopy, and 3D printing. A large-scale dataset of over 6,000 UCNP emission spectra was generated and used to train a GNN model. Gradient-based optimization identified structures with a predicted emission 6.5 times higher than any UCNP in the training set under 800nm illumination. This work reveals new design principles for UCNPs and provides a roadmap for DL-based inverse design of nanomaterials.